Data Analytics
Data analysis or data analytics is the process of examining, cleansing, transforming, and modeling data with the goal of uncovering valuable insights, drawing conclusions, and supporting decision-making. It involves the use of various statistical, mathematical, and computational techniques and tools to identify patterns, trends, correlations, and relationships within a dataset. Data analytics is widely used in fields such as science, industry, government, and research to extract meaningful knowledge that can help organizations optimize their operations, improve efficiency, and gain competitive advantages. Data analysis professionals often employ specialized software and advanced techniques to interpret large volumes of data and present the results in a comprehensible manner for stakeholders.
Data analysis is crucial for businesses in today's digital era, where enormous amounts of data are generated daily. This data contains valuable information that can help businesses better understand their operations, customers, and market. Instead of relying on assumptions or intuition, companies can use data analysis to make evidence-based decisions.
One of the most fundamental ways businesses use data analysis is to understand their customers. By collecting and analyzing data on customer behavior, preferences, and opinions, companies can create detailed customer profiles. This allows them to personalize their products and services to meet their customers' specific needs, which in turn improves customer satisfaction and fosters brand loyalty.
Moreover, data analysis is crucial to understand market trends and consumer preferences. By analyzing data on past purchases and market trends, companies can anticipate future demand and adjust their strategies accordingly. This is especially important in industries where tastes and trends change rapidly, such as fashion or technology.
Another key aspect is operational efficiency. Companies can analyze data to identify areas where they can improve efficiency, reduce costs, and optimize processes. This may involve identifying bottlenecks in the supply chain, optimizing delivery routes, or effectively managing inventory.
Data analysis is also essential for fraud prevention and security. Companies can use advanced algorithms to analyze patterns and detect unusual activities that may indicate fraud. This is particularly relevant in financial and e-commerce sectors where fraud can be a significant threat.
What is data analysis?
Data analysis is the essential process by which data is transformed into knowledge, intelligence, and business insight. In a business context, this process is used to thoroughly understand the state of the business, customer behavior, competition dynamics, and market trends. It is also employed to identify problems, errors, or ineffective strategies, define target customers and buyer profiles, as well as test and discard theories. Its primary function is to support the process of making data-driven decisions, providing a solid, reliable, and credible foundation on which to base these decisions.
For data analysts to extract value from data, it is crucial that the data has undergone a thorough process. This process involves data collection, integration, transformation, normalization, consolidation, and data quality assurance. It is similar to the idea that a carpenter would never try to build a table from an unprepared tree trunk; similarly, data analysts cannot analyze data that is disorganized, lacking proper formatting, or containing errors.
Furthermore, for data analysis to be adequate and efficient, it must be conducted by experts in the field, such as data scientists, analysts, and engineers. Prior to analysis, it is essential to formulate business questions that need to be answered and establish the business objectives to be achieved. In other words, data analysis must be integrated at the highest level of the business strategy.
Today, data analysis should be at the core of every organization. Data is no longer solely the responsibility of the IT department; it is closely linked to all corporate business intelligence strategies and actions, as well as the overall decision-making process. Just as a carpenter cannot build a table without the right materials, a driver cannot operate a vehicle without gasoline, and a company director cannot make informed decisions without data.
In this sense, data should not be seen as simple materials stored in a drawer. Nowadays, it is essential for data to be an integral part of the global business culture. This implies that companies must actively work to build a data-driven culture and ensure that data analysis is employed in all business departments, not just IT. This complete integration of data into the company's structure is crucial for thriving in the contemporary business environment.
Data analytics in business
Data analysis, also known as data analytics, has become an essential element in today's business world. Business leaders need a deep understanding of their operations, market, competition, and customers to make informed decisions and guide their businesses towards success.
Despite the abundance of available data, many companies fail to tap into its true potential. According to Forbes research, even though we generate more data than ever before, businesses are becoming less data-focused.
As astronomer and writer Clifford Stoll once said, "Data is not information, information is not knowledge." To turn data into valuable information, it is crucial to integrate and consolidate the data. Furthermore, to extract meaningful insights, the data must undergo thorough and conscious analysis. This process allows us to use the data in our business intelligence strategies, deciphering what we truly need to know and transforming it into actionable insights.
Data analysis is not only crucial for decision-making but also supports numerous business routines. From enhancing the customer experience to optimizing processes and uncovering new business opportunities, the applications of data analysis are virtually limitless in the business environment. It enables us to interpret the business reality, gain a global view of the business, foster critical thinking, and optimize activity monitoring.
In an increasingly uncertain and competitive market, data is the driving force behind any organization. Despite this reality, many organizations struggle to effectively harness their data. To achieve this, it is imperative to have data analysts and develop a comprehensive data strategy that encompasses all areas of the organization.
In summary, in today's business world, relying on data is not just a trend but a necessity. Companies that understand and effectively utilize their data are better equipped to thrive in the future, adapting to changes and making informed decisions that will drive long-term success.
What is the purpose of data analysis in a company?
Data analysis in the business environment is incredibly versatile and its applications are so varied that it is impossible to list them all. However, here are some of the most common and essential ones:
Data analysis in the business environment is incredibly versatile, with applications so varied that listing them all is practically impossible. Nevertheless, here are some of the most common and essential uses:
Interpreting Business Reality: Analyzing data provides a deep and accurate understanding of the current state of the company and all its areas.
Gaining a Global Vision: It enables obtaining a complete and reliable perspective of the business as a whole, including all its facets and operations.
Stimulating Critical Thinking: It encourages reflection and profound analysis, enhancing the ability to assess situations and make informed decisions.
Generating Ideas and Value: It facilitates the extraction of innovative and valuable ideas, offering a deeper understanding of data and its relevance to the business.
Enhancing Business Monitoring: It allows for more precise and real-time monitoring of business activities, identifying areas of improvement and success.
Promoting Collaboration between Departments: It facilitates interdepartmental collaboration by providing shared and understandable data for all teams.
Process Optimization: It identifies inefficiencies and areas for improvement in business processes, enabling their optimization to increase productivity.
Accelerating Work Pace: By providing relevant information quickly, it helps streamline operations and decision-making processes.
Identifying Errors and Improvement Areas: It uncovers errors and weaknesses in processes, as well as areas where significant improvements can be made.
Predicting Future Scenarios: Using forecasting techniques, it assists in predicting possible future scenarios based on historical patterns and current data.
Deep Customer Understanding: It provides a detailed understanding of customer behavior, preferences, and needs, aiding in personalizing strategies.
Defining Target Customers and Buyer Personas: It facilitates the identification and creation of detailed profiles of target customers and buyer personas for more effective marketing strategies.
Identifying Business Opportunities: It analyzes market data to identify niches and opportunities, informing about potential directions for business growth.
Enhancing Customer Experience: It helps understand customer interactions with the business, enabling personalized improvements for an optimal customer experience.
Optimizing Existing Markets: It allows adjustments to marketing and sales strategies to maximize performance in markets where the company is already present.
Realigning Business Strategies: It facilitates agile adaptation of business strategies in response to changes in the market or customer demands.
Adapting to an Uncertain and Unstable Market: It offers vital information to adjust strategies in volatile markets, ensuring business flexibility and adaptability.
Increasing Return on Investment (ROI): By enabling more effective and efficient strategies, it contributes to maximizing the return on investment across all business operations.
Risk Reduction: It helps identify and mitigate potential business risks by providing solid information for informed decision-making.
Ultimately, data analysis becomes an invaluable compass for companies, guiding them toward smarter decisions, more effective operations, and a sustainable competitive advantage in a dynamic and challenging business world.
How to extract value from data?
Turning data into business value is a meticulous process, much like transforming a raw stone into a diamond. Initially, data is like rough stones: they possess intrinsic value, but this value is minimal in its original format and needs to be unearthed and polished to be useful.
1. Data Extraction: Data resides in various sources, from analytical platforms to emails and social media. At Bismart, we have the ability to extract data from any relevant source, including structured and unstructured databases, legacy corporate applications, and files in various formats.
2. Data Ingestion and Integration: Once extracted, the data needs to be unified and processed. Data integration is essential, ensuring that the data is understandable and uses a common language. This process involves classifying and cleaning up useless data, as well as removing duplicates. Tools like Azure Purview make this task easier, allowing data scientists to create a real-time global map of the company's data landscape.
3. Data Transformation: The classified data undergoes the process of "data wrangling," where it is cleaned and transformed for use in business intelligence projects. Tools like Azure Data Factory and Azure Databricks enable data preparation for analysis. These platforms facilitate the transformation, organization, and analysis of data, preparing it for use in business intelligence strategies.
4. Export and Consumption of Data: The prepared data is exported to specific destinations, such as data warehouses or analysis tools like Power BI. It can also be used in artificial intelligence platforms, machine learning, or transformed into various formats as needed. Data export involves processes like edge computing, bringing computation and data storage closer to the source to accelerate the process.
From Stone to Diamond: Transforming Data into Business Value: At Bismart, we specialize in transforming data into actionable business insights and value. Each step in this process is crucial, and we tailor our approach to meet the unique needs of each client. Our goal is to help you make the most of your data, turning it into business intelligence and tangible value.
Data as a Product (DaaP)
The concept of "Data as a Product (DaaP)" has gained significance within the context of "data mesh", where data is considered an essential product. Treating corporate data as a product is crucial for building a strong enterprise data mesh, a key principle in the data mesh paradigm.
While the idea of considering datasets as products is not new and has been practiced in early data warehouses, the relevance of "Data as a Product (DaaP)" has increased with the popularity of flexible data architectures such as data mesh.
According to IDC, by 2026, only 10% of the data generated annually will be completely new, while the remaining 90% will be reused from existing data. This reality underscores the need to treat data as a product in itself, not just as a tool to build other data products.
Although the term "Data as a Product (DaaP)" was coined by Zhamak Dehghani, there is confusion regarding the difference between "data as a product" (DaaP) and "data products". To clarify, DaaP refers to the perspective of considering data as reusable entities, providing information when needed for business processes or specific analysis and strategic decision-making.
In this approach, data must meet fundamental characteristics: being easily discoverable, secure, addressable, understandable, and reliable. The Chief Data Officer plays a crucial role in ensuring that data assets meet these criteria.
In summary, "Data as a Product (DaaP)" involves applying product development thinking to datasets, ensuring they meet high-quality standards, transforming them into valuable and reusable products for the entire organization.
What is the difference between Data as a Product (DaaP) and a data product?
The term "Data as a Product (DaaP)" has often been confused with "data product," leading to some confusion between these two terms, even though they do not mean the same thing.
If we refer to the first official definition of a "data product," coined by DJ Patil in his book "Data Jujitsu: The Art of Turning Data into Product" (2012), a data product is "a product that facilitates achieving a final goal through the use of data." In other words, it is any product that utilizes data to accomplish a specific objective. For example, an online newspaper could be considered a data product if it dynamically selects news based on a user's browsing history.
In 2018, Simon O'Regan provided specific examples of data products in his article "Designing Data Products," categorizing them into types such as raw data, derived data, algorithms, decision support, and automated decisions.
In summary, a "data product" is a general concept that includes any product driven by data. On the other hand, "Data as a Product (DaaP)" is a mindset that involves treating data as a product.
Concrete examples of data products to understand the difference are:
Data Warehouse: It is a data product that combines raw and derived data, serving as a decision support system.
Business Dashboard: It visually represents key performance indicators (KPIs) of a company, functioning as a data product in the decision support system category. The interface it provides for accessing information is a visualization.
Recommended Restaurants List: It is a data product that uses an automated system to make decisions, providing specific recommendations for a particular user.
Autonomous Car: It can be considered a data product as it makes decisions automatically based on collected data, being a data product in the automated decision-making category.
The key features of "Data as a Product (DaaP)"
How does the idea of "data as a product" materialize? Data in the form of a product includes not only the data itself but also the associated code and the infrastructure necessary for its execution.
Authorities in the field of data highlight a series of features that data and its management must fulfill to be considered "Data as a Product (DaaP)."
Data as a Product (DaaP) must have the following characteristics:
Accessible
Having a search engine that allows users to register datasets and request access when needed is crucial. Initially, this could involve simply having a list of internal datasets, progressively building and improving from there.
Addressable
The availability of easily locatable datasets significantly enhances productivity. Analysts and data scientists can independently search for and use data, and data engineers are not constantly interrupted by queries about specific data locations.
Self-Description and Interoperability
In a world where companies accumulate more and more data, datasets must include clear metadata and follow consistent naming guidelines to promote interoperability. It is essential for datasets to include detailed descriptions, such as data location, source, sample data, information about updates, and input prerequisites.
At Bismart, we have a solution that self-documents Power BI datasets, providing functional and business descriptions. Power BI Data Catalog enables appropriate data usage and allows users to generate reports without technical assistance.
Reliable and Secure
Ensuring data quality periodically and automatically is essential to meet reliability expectations. Data custodians must respond to quality assessment results, which should be conducted both when entering and consuming data. Additionally, it is important to provide context about data quality to consumers.
At Bismart, we offer a solution to support an organization's data quality, evaluating, validating, and documenting data, ensuring an optimal level of quality.
Finally, registered and evaluated datasets should not be automatically available to everyone. It is advisable for users to request access individually for each dataset, and dataset custodians should approve or deny access.
In summary, the concept of "Data as a Product (DaaP)" is essential for building a robust corporate data mesh. Treating data as a product involves understanding its value as reusable elements for providing information and making strategic decisions. Unlike data products, data as a product focuses on ensuring fundamental features such as ease of detection, security, addressability, understanding, and reliability.
How to Define a Data Analysis Strategy?
Currently, the success of any business is closely tied to the adoption of a modern and effective data analysis strategy. Although most companies recognize the value of data and analytics, developing a data strategy and truly capitalizing on its potential can be overwhelming at first. In this context, we will explore the four essential steps to develop a data strategy that truly drives business value.
Are companies fully leveraging the value of data?
Despite advancements, not as much as they could be. According to Forbes, fewer companies are adopting a data-driven mindset. Surprisingly, 62.2% of companies have yet to establish a fully data-oriented culture, and astonishingly, 95% of enterprise data remains unused.
In a previous article, we analyzed why companies are still hesitant to adopt a data-driven mindset. We emphasized the importance of business leaders understanding the intrinsic value of data and developing a comprehensive strategy that encompasses crucial areas such as data analysis, data governance, and data quality. Additionally, it is essential for all levels and employees of the company to effectively work with data, beyond just the subject matter experts.
If you want to discover the four essential steps to create a solid data strategy, we invite you to check out our free ebook, "How to Create a Data Strategy to Fully Leverage the Business Value of Data."
How to establish a data strategy to make the most of your company's resources?
The transformation begins at the top of the organization. The first step to implementing an effective data strategy and fostering a data-driven culture is for leaders to understand the intrinsic value of information and ask the right questions:
- What data do we need to make informed decisions?
- What information is crucial for us?
- How can we enhance our offerings for our customers with this data?
- How can we optimize our internal processes with the available information?
- How can we use this data to transform our business?
Asking these questions is a significant step forward, but answering them is even more crucial. The third step involves implementing a comprehensive analytical system and providing training on data comprehension throughout the organization.
Ultimately, a data strategy must address data quality, data governance, and data literacy. These three pillars are essential to ensure the success of any investment in data and analytics.
How to create a strong and well-conceived data strategy? According to our experts, the most crucial aspect is establishing a solid strategic foundation. This means that the techniques should align with the strategy, not the other way around. While deciding on which information and analytical systems to invest in is vital, the fundamental focus should be on the strategic approach.
Every data strategy must stem from the company's mission and key objectives. Business leaders must identify which outcomes are priorities and translate them into specific business objectives that will guide the data strategy. Without a clear approach, even the most advanced technological investments may not yield the desired results.
Furthermore, data strategies should be based on four fundamental premises:
- Data Governance
- Data Analytics
- Data Literacy
- Data Quality
In summary, in a modern and digital company, data should play a central role. Developing a goal-oriented data strategy that encompasses the four essential pillars (strategy, literacy, governance, and data quality) is the way forward to maximize the potential of your data.
Data analytics to consolidate a data-driven culture
Nowadays, every company, regardless of its size, has access to data. Some organizations have taken advantage of this data to gain valuable insights into their internal state, customer interactions, and operational performance, ultimately improving decision-making and optimizing processes. However, there are still many companies that are not effectively utilizing their data. It's not so much that these companies are unaware of the potential value of business intelligence, but rather that they lack a data-driven culture ingrained in their organization. This lack of culture hinders the implementation of analytical technologies.
Simply having data and recognizing its value is not enough. Companies must intelligently use data to make informed decisions about their business and processes. In other words, it is crucial to know how to interpret the data.
Innovative companies use data for prediction, providing a solid foundation for future decision-making. Data prepares the company to face what lies ahead. To achieve this, employees need to have knowledge in data management to participate in decision-making at all levels and discover new opportunities.
To foster data literacy, organizations must cultivate a work culture that promotes the use of data and supports evidence-based decision-making. Additionally, it is essential to stimulate curiosity and critical thinking, especially in relation to data. This requires the right combination of technology and people. Data not only facilitates daily work and supports decisions but also opens up new possibilities through trend identification. By involving the entire team in this process of innovation, from a data-driven perspective, each member can understand their impact and appreciate the advantages that a change in the corporate culture can offer.
Top data analysis tools
Currently, the field of data analytics tools has exponentially expanded, leading to a wide variety of options. Choosing the right tool has become a complex task, considering factors such as performance orientation and user-friendliness. Moreover, data analysis is no longer confined to a single process; it is now intrinsically linked to data integration, data consolidation, and data quality.
Here are some data analysis tools that are incredibly useful for managing data effectively:
Microsoft Power BI: Power BI, created by Microsoft, is a highly popular analytical tool that offers interactive visualizations and seamlessly integrates with other Microsoft tools. It can connect to more than 60 data sources and is accessible even for users with limited technical knowledge.
R Programming: R is a powerful analytical tool primarily used for data modeling and statistics. It is user-friendly and highly versatile, surpassing many other tools in terms of performance and data capacity.
SAS: SAS is a programming language that simplifies data manipulation. It is manageable, accessible, and can analyze data from any source. It is widely used for profiling clients, predicting behaviors, and optimizing communication with them.
Python: Python, an open-source tool, is easy to learn and has libraries for machine learning. It is compatible with various platforms and databases, such as JSON and MongoDB.
Excel: Excel is a basic yet versatile analytical tool used in almost every industry. While fundamental, it also offers advanced options for business analysis, such as DAX functions and automatic relationships.
Don't miss our post: Excel vs Power BI
Tableau Public: Tableau Public is free software that connects various data sources and creates real-time dashboards and visualizations. It is ideal for analyzing and visualizing high-quality data.
Rapid Miner: Rapid Miner is a comprehensive tool for data science. It offers predictive analysis and data mining without programming. It can integrate with a variety of data sources and generate analyses based on real data.
Apache Spark: Apache Spark is a large-scale data processing engine. It is highly popular for developing machine learning models and data pipelines. It also has a library called MLib that offers a variety of advanced algorithms.
Qlik: Qlik is a comprehensive suite of platforms for data analysis, integration, and programming. It includes notable tools like QlikSense and QlikView, which specialize in analysis and business intelligence. This solution is deployed in a hybrid cloud and offers a complete range of integration and analysis processes, including functions for automating the data warehouse.
With Qlik, you can integrate and transform data, create visualizations and dashboards from analysis, and even delve into augmented analytics. Like many of the technologies mentioned earlier, Qlik is an excellent choice for turning data into intelligence and facilitating business decision-making. It provides companies with the ability to analyze multiple data sets from various sources, offering a global view of company information.
Moreover, Qlik enables detailed statistical analysis through data, as well as the creation of dynamic charts, presentations, and impactful visualizations.
QuickSight:
QuickSight is Amazon's cutting-edge analysis and business intelligence tool. It is a cloud-based service that seamlessly integrates with various data sources such as AWS, SaaS, and Excel.
Its primary objective is to empower decision-makers to explore data and interpret information in a visually appealing and user-friendly manner. Despite its intuitive approach, QuickSight also offers advanced capabilities, including the ability to perform machine learning. Similar to Power BI, it enables the sharing of analyses and reports, facilitating collaborative analysis.
Data Analytics with Power BI
Microsoft Power BI is a leading tool for data analysis, business intelligence, and reporting. It has been recognized by Gartner's Magic Quadrant this year and has maintained its position in 2020. Beyond the endorsement of a prominent global technology consulting firm, Power BI is preferred by professionals due to its strategic approach designed to meet business needs.
This powerful tool allows data scientists and analysts to transform data sets into engaging and interactive reports, dashboards, and visualizations. This enables precise tracking of business activity and strategic decision-making.
As partners of Microsoft Power BI, at Bismart, we have been working with this suite of services for many years, developing customized solutions for our clients. Data analytics with Power BI not only facilitates the discovery of insights but also democratizes data through its robust visualization capabilities. This creates a high-quality internal knowledge base, empowering executives to make data-driven decisions.
Power BI simplifies the work of consultants and data analysts by making data connection, transformation, and visualization easy and accessible.
The benefits of utilizing Power BI for data analysis:
Data Connection and Integration: Power BI stands out for its extensive data connectivity. It connects to multiple tabular databases and integrates with various corporate tools and systems, enabling quick and easy data import, export, dashboards, and reports.
Data Visualization: Power BI is a comprehensive data visualization platform. It offers a variety of Microsoft-validated Power BI visuals and allows the creation of custom visuals. The tool is regularly updated with new visuals and can enhance its capabilities with tools like Zebra BI, maximizing options for both Excel and Power BI.
Advanced Analytics: Power BI goes beyond traditional data analysis in tools like Excel, offering advanced analytics options. It enriches business data by ingesting, transforming, and integrating data services embedded in other Microsoft suite tools.
Data Governance: Data governance is crucial for anyone working with data, especially in the business environment where data is invaluable. Power BI includes features that promote data control, authority, and management. However, some organizations might require specialized data governance solutions that integrate with Power BI.
Data Exploration: Power BI offers extensive options for in-depth data exploration and query automation. It facilitates insights discovery from data and is ideal for working with the top-down methodology, simplifying the process of discovering patterns and trends.
User Experience and Design: Designed as a business tool, Power BI is accessible to users with business profiles. While it is essential for data analysts and BI consultants, its business-oriented approach ensures excellent usability and an intuitive user interface. Additionally, Power BI allows customization of reports to align with the organization's corporate image and automates the process through predefined themes applicable to all reports.
In summary, Power BI has become a leading technology in the data analysis and BI market due to its business-oriented approach and robust capabilities. It helps companies transform data into intelligence, facilitating informed and strategic decision-making.
Data Analytics vs Data Science
In the realm of data, there is a common confusion surrounding the terms data science and data analytics. These two professional areas are closely linked but generally serve different functions.
Both data science and data analytics utilize statistics, mathematics, and programming, but with distinct purposes. Professionals in these disciplines possess different skills and knowledge.
What is Data Science? Data science, or the science of data, is a discipline related to data that primarily focuses on the processing of large volumes of data collected by companies. Data scientists transform raw data into understandable and usable information for business actions. This includes activities such as machine learning, deep learning, data integration, and the development of mathematical algorithms. They also ensure that data is usable and transformed into valuable information for strategic decision-making.
Data science can be divided into three sub-disciplines: data preparation, data cleaning, and data analysis. Data analytics falls within the realm of data science.
What is Data Analytics? Data analytics is a branch of data science that focuses on data analysis. Data analysts are experts in analyzing data using analytical tools and business intelligence. Their task is to identify trends, transform data into metrics and performance evaluations, identify relevant aspects, and draw conclusions.
In today's business environment, data analysis is essential for optimal organizational functioning. Data analysts transform data into information, and this information into business insights that aid in strategic decision-making.
The key difference between data analytics and data science lies in their approach. While data science takes a global perspective and encompasses any action related to data processing, data analytics focuses on data analysis to obtain business insights and solve existing problems.
In summary, data analytics is a sub-discipline of data science that focuses on detailed data analysis to assist business leaders in making informed and strategic decisions. Each of these disciplines has its own set of skills and specific roles in the world of data.
What is Advanced Analytics?
In today's business world, data analysis is essential for understanding operations, gaining valuable insights into competitors, and defining more effective customer strategies. However, with the growing volume of generated data, there is a need for more advanced analysis techniques, leading to the concept of "Advanced Analytics."
Definition of Advanced Analytics: Advanced Analytics, as the name suggests, refers to a type of data analysis that incorporates advanced techniques. These techniques go beyond traditional data analysis, allowing for the discovery of hidden patterns, predicting future outcomes, and providing deeper strategic insights. One of the distinctive aspects of Advanced Analytics is the use of artificial intelligence capabilities, such as sophisticated algorithms and complex mathematical models, for prediction.
While conventional data analysis methods focus on describing and analyzing past events, Advanced Analytics aims to understand why certain events occurred and what is likely to happen in the future. Predictive analysis is a key branch of Advanced Analytics, but it is not the only one.
Types of Advanced Analytics:
-
Predictive Analysis: Predictive analysis uses statistical techniques, machine learning, and deep learning to predict future events or behaviors based on historical data. By applying predictive models, companies can anticipate trends, make proactive and strategic decisions, and foresee customer behavior.
-
Data Mining: Data mining involves discovering hidden patterns and relationships within large datasets. Using advanced algorithms like clustering, decision trees, grouping, or anomaly detection, organizations can gain valuable insights into customer behavior, identify improvement opportunities, and optimize business processes.
-
Text Analytics: With the growth of unstructured data, such as emails and social media, Text Analytics has become crucial. This technique allows for the analysis of large amounts of text, identifying sentiments, opinions, and relevant topics.
-
Social Media Analysis: Social Media Analysis focuses on examining data from social platforms to discover interaction patterns and user behavior. It helps organizations better understand their audience, adapt their marketing strategies, and make data-driven decisions based on online feedback.
-
Big Data Analysis: Big Data analysis deals with managing and analyzing large volumes of structured and unstructured data. This discipline uses techniques and tools to process, store, and analyze data on a massive scale. Big Data analysis enables organizations to extract relevant information from diverse sources and leverage it for strategic decision-making, gaining a competitive advantage.
In summary, Advanced Analytics represents a step forward in data analysis, employing advanced techniques to provide deep strategic insights and predict future events. This advanced approach assists companies in making more informed and strategic decisions.
How Does Advanced Analytics Transform Businesses?
The effective implementation of advanced techniques in analyzing business data, known as Advanced Analytics, offers several significant benefits to organizations.
1. Data-Driven Decision Making: Advanced Analytics provides precise and relevant information for informed decision-making. By combining structured and unstructured data and applying sophisticated analytical techniques, businesses can make more conscious and accurate strategic decisions.
2. Competitive Advantage: By harnessing the capabilities of Advanced Analytics, organizations gain a significant competitive edge. Understanding customers better, predicting market trends, and optimizing internal processes enable businesses to anticipate changes and quickly adapt to market demands.
3. Resource Optimization: Advanced Analytics helps businesses optimize their resource usage. By identifying inefficiencies and areas for improvement, organizations can reduce costs, enhance operational efficiency, and maximize performance.
4. Innovation and Opportunity Discovery: Advanced Analytics enables the discovery of new business opportunities and fosters innovation. By analyzing large volumes of data and uncovering non-obvious patterns, companies can identify market niches, anticipate customer needs, and develop new solutions.
5. Predictive Analysis and Forecasting: Predicting trends is one of the significant benefits of Advanced Analytics for businesses. Through this analysis, organizations can anticipate market changes and quickly adapt to customer demands. Predictive analytics not only aids businesses in making informed long-term decisions but also allows them to stay ahead of the competition and develop new solutions as market needs arise.
In an increasingly data-driven business environment, Advanced Analytics has become a crucial component of corporate data analysis. By enabling deeper analysis, predicting future events, and generating strategic insights, Advanced Analytics empowers organizations to make informed decisions and gain a significant competitive advantage. In a context where data analytics plays an increasingly central role in the corporate environment, advanced analytics is essential for businesses to unlock the true value of their data and explore new business opportunities.
Revolutionizing Data Analysis with Text Analytics
Text Analytics technologies have transformed how businesses handle unstructured data, specifically written text. These systems are integral to artificial intelligence, utilizing complex algorithms to decipher patterns in texts that would otherwise be difficult to comprehend.
The relevance of this capability is undeniable, considering that approximately 80% of crucial information for organizations is hidden in unstructured data, predominantly in the form of text. Fortunately, there are numerous Text Analytics systems available today. However, not all are equal. Understanding their functionalities and differences is essential.
These systems primarily operate under two methods: taxonomy and folksonomy. Taxonomy requires prior organization of information through predefined labels to classify content. On the other hand, folksonomy is based on natural language tagging, allowing significant adaptability and flexibility in the classification process.
What does Natural Language Processing (NLP) entail? Natural Language Processing (NLP) is a branch of artificial intelligence (AI) that focuses on the relationship between computers and human language. Its main task is to teach computers to understand, interpret, and generate human language in a meaningful and useful way.
The fundamental purpose of NLP is to serve as a bridge between human communication, which often involves unstructured and ambiguous language, and the structured and precise nature of computer languages. Thanks to NLP, machines can process, analyze, and extract information from vast amounts of textual data, emulating human skills in this aspect.
What are Large Language Models (LLM)? Large Language Models (LLM) constitute a category of artificial intelligence models capable of understanding and generating human language. These models are based on deep learning techniques and are trained with extensive textual datasets to develop a deep understanding of language patterns and structures.
What is the difference between Taxonomy and Folksonomy? Taxonomy is a hierarchical classification system where contents are categorized into a structured and predefined set of categories. It follows a top-down approach, with categories and subcategories determined by experts or administrators.
In contrast, Folksonomy is a user-generated classification system where contents are tagged and categorized by users themselves. It follows a bottom-up approach, as users assign their own tags based on their understanding and context, without a predefined structure.
Let's now explore some of the most common applications of these Text Analytics technologies, highlighting their versatility across various business domains.
Examples of Text Analytics
Text Analytics systems play a crucial role in various aspects of our digital life, revealing patterns and organizing information significantly. Here are five real examples of how this technology revolutionizes our interaction with data:
Hashtags and Social Media Tags: Have you ever used hashtags on social media? These simple symbols, like #DataAnalytics, are recognized by Text Analytics algorithms. These algorithms organize content, whether texts, images, or videos, based on these tags, facilitating the search and classification of content on digital platforms.
Google and its Search Algorithm: Google, the giant of search engines, uses Text Analytics algorithms to find and organize content based on keywords. It not only interprets text directly from websites but also text in files, videos, and documents, providing accurate and relevant results to user queries.
Flickr and Folksonomy: Flickr, a popular platform for sharing photos, leverages Text Analytics systems based on folksonomy. Users tag their images with descriptions and locations. These tags not only organize the images but also reveal trends and relevant tags at any given time.
Bismart Folksonomy Text Analytics in the Medical Field: In the healthcare sector, information is abundant but often disorganized. Bismart Folksonomy Text Analytics solution uses artificial intelligence to process unstructured medical data, such as patient medical histories and symptoms. This system generates tags based on word frequency in the text, allowing efficient classification and analysis of massive medical data.
Search Engine Optimization (SEO) Optimization: SEO analysis tools use Text Analytics to analyze the content of web pages. They evaluate patterns in the text, such as tags and descriptions, to improve a page's ranking on search engines.
In summary, Text Analytics systems are transforming how we interact with data, from our social media interactions to medical research and web optimization. These examples illustrate how this technology is fundamental in organizing and understanding data in an increasingly complex digital world.
The best text analytics tools
SAS
SAS is a powerful software tool designed to extract valuable information from unstructured data, including online content such as books and comment forms. In addition to facilitating the machine learning process, this software can automate the reduction of generated topics and rules, allowing you to observe how they evolve over time and adjust your approach for better results.
QDA Miner's WordStat
QDA Miner offers various capabilities for analyzing qualitative data. Using its WordStat module, the program can analyze texts, perform content and sentiment analysis, and analyze web pages and social media for business intelligence. This module includes visualization tools that aid in interpreting the results, and WordStat's correspondence analysis helps identify key concepts and categories in the analyzed text.
Microsoft Cognitive Services Suite
Cognitive Services provides a robust set of artificial intelligence tools to create smart applications with natural and contextual interactions. While not exclusively a text analysis program, it integrates elements of textual analysis into its approach to understanding speech and language. One of these elements is the intelligent language understanding service, designed to assist bots and applications in comprehending human input and communicating with people naturally.
Rocket Enterprise's Search and Text Analytics
Security is a primary concern for companies dealing with large volumes of data (Big Data), and Rocket's tool addresses this in its text analytics solution. In addition to its focus on security, this tool stands out for its user-friendly interface, allowing teams to find information quickly and easily, especially beneficial for those with limited technological experience.
Voyant Tools
Voyant Tools is an accessible application for text analysis on web pages, particularly popular among academics working in digital humanities worldwide. While it doesn't delve deeply into textual analysis, it offers a straightforward interface and performs various analysis tasks. It can quickly analyze a web page and provide visualizations of the data in the text within seconds.
Watson
Watson, the renowned IBM system that defeated Jeopardy! champion Ken Jennings, features Watson Natural Language Understanding. This technology uses cognitive intelligence to analyze text, including sentiment and emotion evaluation, demonstrating its excellence in high-quality text analysis.
Open Calais
Open Calais is a cloud-based tool focused on content tagging. Its strength lies in recognizing relationships between different entities in unstructured data and organizing them accordingly. Although it doesn't delve into complex sentiment analysis, it is effective in managing unstructured data and converting it into a well-organized knowledge base.
Folksonomy Text Analytics
Bismart's intelligent Folksonomy software uses tags based on generative artificial intelligence (IAG) and Large Language Model (LLM) machine learning models to filter unstructured data files and locate specific information. Notably, you don't need to define tags and categories manually; the system can be programmed and restructured in real-time for different purposes, making it versatile and fast, ideal for collaborative projects.
Predictive Data Analytics
Predictive data analytics is a branch of data analysis that harnesses algorithms, statistical techniques, and machine learning models to uncover patterns and trends in historical data and predict future events or outcomes. In essence, predictive data analytics uses past data to make predictions about future events.
This approach involves using historical data to develop predictive models that can inform decision-making and anticipate likely outcomes. By employing advanced algorithms and techniques, predictive data analytics can forecast behaviors, identify risks, uncover opportunities, and optimize processes.
For example, in the realm of marketing, predictive data analytics can be used to forecast customer behavior and personalize marketing strategies accordingly. In the financial sector, it can be utilized to predict market trends and make more informed investment decisions. In the healthcare sector, predictive data analytics can help forecast disease outbreaks and allocate resources more efficiently.
In summary, predictive data analytics is a powerful tool for transforming historical data into valuable insights that enable accurate predictions about future events and informed decision-making based on those predictions.
Companies require predictive analysis tools to anticipate future situations, adapt to the market, and optimize long-term strategies. Within predictive analysis, there are two main approaches: classification models and regression models. This is not science fiction; it is business intelligence. Predictive analysis models and algorithms have become a crucial part of artificial intelligence and are widely utilized by businesses.
In an increasingly complex and information-saturated world, the ability to predict future events becomes crucial. In business, predictive analysis has become a necessity, although many companies use it daily without realizing it. Artificial intelligence algorithms, machine learning, and deep learning have become indispensable business tools.
The applications of predictive analysis are diverse, ranging from predicting customer behavior and competition to forecasting inventories and more. Short-term, medium-term, and long-term predictions are fundamental in data analysis strategies and business intelligence.
Types of predictive analysis
There are two fundamental approaches in predictive analysis: classification models and regression models, both integrated into supervised machine learning.
Classification models are used to assign a category or class to a new variable, such as predicting whether a customer will purchase a product again. These models are especially useful in binary situations, like predicting if production will decrease or if the price of electricity will increase.
On the other hand, regression models are more complex and are employed to forecast continuous values, such as inflation in a country or the profits generated by a company in the next year. Unlike classification models that offer categorical answers, regression models predict numerical outcomes and have the ability to handle a wide range of possibilities.
In addition to these basic approaches, there are various techniques applied in predictive analysis. For example, regression analysis relates variables to each other, which can be logistic (for categorical variables) or linear (considering dependent and independent variables). Decision trees, on the other hand, are multi-branch structures that split into different variables, and neural networks mimic the structure of the human brain, connecting simple elements in multiple layers.
Although these methodologies may seem complex, they have become common tools in the business world to anticipate events and make informed decisions, even if their internal workings are not fully understood.
Classification vs Clustering
Both classification and clustering are methods used in the field of machine learning (also known as "aprendizaje automático" in Spanish) to identify patterns. Despite their similarities, they differ in their approach: classification relies on predefined categories to assign objects, while clustering groups objects based on similarities, creating distinct sets from other groups. These sets are referred to as "clústeres" or "clusters." In the realm of machine learning, clustering falls under unsupervised learning, which means we only have input data (unlabeled) and must extract information without knowing the expected output beforehand.
Clustering is used in business projects to identify common characteristics among customers and thereby tailor products or services, similar to customer segmentation. For example, if a significant percentage of customers share certain traits (age, family type, etc.), specific campaigns, services, or products can be justified.
On the other hand, classification falls under supervised learning. This means we know the input data (labeled in this case) and the possible outputs of the algorithm. There are binary classifications for problems with categorical responses (such as "yes" or "no") and multiclass classifications for problems with more than two classes, where responses are more varied, such as "excellent," "average," or "insufficient."
Classification has various applications, from biology to the Dewey Decimal Classification for books, as well as in detecting unwanted emails (spam). These techniques enable businesses to better understand their data and make informed decisions.
Examples
One notable example of the application of clustering algorithms is Netflix's recommendation system. While the company remains tight-lipped about specific details, it is known that there are approximately 2,000 clusters or communities with similar audiovisual preferences. For instance, Cluster 290 includes individuals who enjoy series like "Lost," "Black Mirror," and "Groundhog Day." Netflix utilizes these clusters to refine its understanding of viewers' preferences, enabling them to make more accurate decisions when creating new original series.
In the financial sector, classification is widely employed to ensure transaction security. In the era of online transactions and the decline in cash usage, it is crucial to determine the safety of card transactions. Financial institutions can classify transactions as either legitimate or fraudulent by utilizing historical data on customer behavior. This precise classification allows them to detect fraud with great accuracy.
These examples illustrate the significant impact that clustering and classification algorithms have on our daily lives. If this topic has piqued your interest, we recommend downloading our white paper on Big Data, where you will find detailed information about data analysis, artificial intelligence, and much more.
How to Conduct a Data Analysis?
When delving into the world of data analysis, we often focus on the essential tools and technological knowledge that drive this scientific field. Despite their importance, these aspects are ultimately dependent on the methodology of the data analysis process.
Now, let's dive into the six essential steps of the data analysis process, providing examples and examining key points along the way. We will explore how to establish clear objectives for analysis, gather relevant data, and conduct the analysis itself. Each of these steps requires specific skills and knowledge. However, understanding the entire process is crucial for deriving meaningful insights.
It is vital to recognize that the success of a business data analysis process is closely related to the level of maturity in the company's data strategy. Companies with a more advanced data-driven culture can perform deeper, more complex, and more effective data analysis.
Step 1: Define a Specific Objective
The first phase of any data analysis involves defining a specific objective for the research. This translates into clearly establishing what you aim to achieve with the analysis. In a business context, the objective is inherently linked to goals and, as a result, key performance indicators (KPIs).
An effective way to define the objective is to formulate a hypothesis and develop a strategy to test it. However, this step is more complex than it initially seems. The fundamental question that should always guide this process is:
- What business objective are we trying to achieve? or
- What business challenge are we addressing?
This process, although it may seem simple, requires a deep understanding of the business and its goals to be truly effective. It is crucial for the data analyst to have an in-depth understanding of the company's operations and objectives.
Once the objective or problem to be solved has been defined, the next step involves identifying the necessary data and sources. This is where the business acumen of the analyst comes into play again. Identifying relevant data sources to answer the question posed requires extensive knowledge of the company and its operations.
Bismart's Tip: How to Set an Appropriate Analysis Objective? Defining an analysis objective is based on our creativity in problem-solving and our knowledge of the field of study. In the context of business data analysis, it is most effective to pay attention to established performance indicators and business metrics in the field we are exploring. Examining the company's reports and dashboards can provide valuable insights into key areas of interest for the organization.
Step 2: Data Collection in the Data Analysis Process
Once the objective has been defined, it's crucial to create a meticulous plan to acquire and consolidate the necessary data. At this point, identifying the specific types of data required is fundamental, ranging from quantitative data like sales figures to qualitative data like customer opinions.
Furthermore, it's essential to consider the typology of data based on its source:
Primary Data: This category includes information directly collected by your organization or data gathered specifically for the analysis at hand. It encompasses transactional data obtained from the CRM (Customer Relationship Management) system or a Customer Data Platform (CDP). These data sets are usually structured and well-organized. Other sources might involve customer satisfaction surveys, opinions from focus groups, interviews, and direct observational data.
Secondary Data: Secondary data originates from other organizations but is firsthand information collected for purposes different from your analysis. The primary advantage of secondary data lies in its organized structure, which simplifies the analysis process. Moreover, these data sets tend to be highly reliable. Examples include activities on websites, applications, or social media platforms, online purchase histories, and shipping data.
Third-Party Data: Third-party data is compiled and consolidated from diverse sources by external entities. Often, these datasets contain various forms of unstructured information. Many organizations leverage third-party data to generate industry reports or conduct market research.
A specific example of third-party data collection and utilization is illustrated by the consultancy firm Gartner, which gathers and disseminates valuable business data to other companies.
Step 3: Data Cleansing and Preparation in the Data Analysis Process
Once we have gathered the necessary data, it is crucial to prepare them for analysis through a process known as data cleansing or "cleaning." This stage is fundamental to ensure the quality of the data we will work with.
Common tasks in this phase include:
Elimination of Errors and Duplicates: Significant errors, duplicate data, and outliers, common issues when combining data from various sources, are removed.
Discarding Irrelevant Data: Observations that are not relevant to the planned analysis are excluded.
Organization and Structuring: General adjustments are made to rectify typographical errors and discrepancies in design, making data manipulation and mapping easier.
Filling Data Gaps: Significant gaps identified during cleansing are promptly addressed.
It is essential to note that this phase is the most labor-intensive, consuming approximately 70-90% of the data analyst's time. For detailed steps in this stage, we invite you to read our data processing guide.
Bismart Tip: To expedite this process, tools like OpenRefine simplify basic data cleaning, even offering advanced features. However, for extensive datasets, Python libraries like Pandas and specific R packages are more suitable for robust data cleansing, although a strong programming knowledge is necessary for effective use.
Step 4: Data Analysis in the Analysis Process
Once the data has been cleaned and prepared, we enter the most exciting phase of the process: data analysis.
It's important to note that there are various types of data analysis, and the choice largely depends on the analysis's objective. Additionally, there are multiple techniques for conducting analysis, such as univariate analysis, bivariate analysis, time series analysis, and regression analysis.
In a broader context, all forms of data analysis can be classified into the following categories:
Descriptive Analysis: This type explores past events and is typically the first step companies take before delving into more complex investigations.
Diagnostic Analysis: It focuses on uncovering the "why" behind something, seeking the causes or reasons behind an event of interest to the company.
Predictive Analysis: This type concentrates on predicting future trends based on historical data. It is especially relevant in the business realm and is linked to artificial intelligence, machine learning, and deep learning. It enables companies to take proactive measures, such as resolving issues before they occur or anticipating market trends.
Prescriptive Analysis: It is an evolution of descriptive, diagnostic, and predictive analyses. It combines these methodologies to formulate recommendations for the future, going beyond simply explaining what will happen. It provides the most suitable actions based on predictions. In the business context, prescriptive analysis is invaluable for determining new product projects or investment areas, utilizing synthesized information from other types of analysis.
An example of prescriptive analysis is the algorithms guiding Google's self-driving cars. These algorithms make real-time decisions based on historical and current data to ensure a safe and smooth journey.
Step 5 of the Data Analysis Process: Communicating Results
After the analysis is complete and conclusions have been drawn, the final stage of the data analysis process involves disseminating these findings to a wider audience, especially stakeholders in the case of a business analysis.
This step entails interpreting the results and presenting them in an easily understandable manner so that leaders can make data-driven decisions. It is crucial to convey clear and concise ideas, leaving no room for ambiguity. Data visualization plays a vital role in this process, and data analysts often utilize reporting tools like Power BI to transform the data into interactive reports and dashboards that reinforce their conclusions.
The interpretation and presentation of results have a significant impact on the direction of a company. Therefore, it is essential to provide a comprehensive, clear, and concise overview that demonstrates the scientific rigor and factual basis of the extracted conclusions. Additionally, it is also crucial to be honest and transparent, sharing any doubts or unclear conclusions that may arise during the analysis and regarding its results with the stakeholders.
Step 6: Implementation of Results in the Data Analysis Process
The final phase of a data analysis process involves transforming the acquired intelligence into concrete actions and business opportunities.
It is crucial to understand that data analysis does not follow a linear path but is rather a complex process filled with challenges. For instance, during data cleaning, unexpected patterns might surface, leading to new questions and potentially requiring a redefinition of your objectives. Exploratory analysis might reveal data that was previously overlooked. You might even discover that the results of your primary analyses seem misleading or incorrect, possibly due to errors in the data or human mistakes in earlier stages of the process.
Despite these hurdles, it is essential not to be discouraged. Data analysis is intricate, and challenges are a natural part of the process.
In summary, the fundamental stages of a data analysis process include:
1. Definition of Objectives: Establishing the business problem you aim to address. Formulating it as a question provides a structured approach to finding a clear solution.
2. Data Collection: Devising a strategy to gather necessary data and identifying the most promising sources capable of providing the required information.
3. Data Cleaning: Delving into the data, refining it, organizing, and structuring it as necessary.
4. Data Analysis: Employing one of the four main types of data analysis: descriptive, diagnostic, predictive, or prescriptive.
5. Results Communication: Choosing effective means to disseminate ideas clearly and encourage intelligent decision-making.
6. Learning from Challenges: Recognizing and learning from mistakes is part of the process. Challenges encountered during the process are learning opportunities that can transform the analysis into a more effective strategy.
Data Analysis Trends
The year 2020 marked a turning point in many aspects and transformed our perception of the world. In the business realm, one of the most notable changes brought about by the Covid-19 pandemic was the rapid adoption of remote work, pushing companies that were previously reluctant to digitize.
According to strategic consulting firm McKinsey & Company, the pandemic significantly accelerated the process of digitalization and technological development in businesses. Prior to the health crisis, 92% of business leaders surveyed by McKinsey already believed that their business models would not be viable at the pace at which digitalization was advancing. The pandemic only intensified this urgency. McKinsey highlighted that simply speeding up the process is not the solution; instead, successful companies are investing in technology, data, processes, and talent to enable agility through more informed decision-making.
Furthermore, the pandemic motivated organizations to move away from traditional trends in analysis and data, which heavily relied on large historical datasets. Many of these data points are no longer relevant, and the current context of change is seen as an opportunity to drive transformation.
According to Gartner, the key trends in analysis and data in the business sector can be grouped into three areas: more agile data integrations and increased use of artificial intelligence in data analysis, the implementation of efficient business operations (XOps), and the increase in the distribution, flow, and flexibility of data assets. These trends reflect the need for adaptation and the search for more flexible and agile approaches in the modern business world.
Advanced and Ethical Artificial Intelligence: Artificial intelligence (AI) remains a key focus in the business realm. The trend in AI is geared towards enhancing its scalability, responsibility, and intelligence to optimize learning algorithms and expedite evaluation times. Gartner indicates that AI will need to operate with less data, as historical data might become irrelevant. Additionally, security measures and data compliance will be intensified to promote ethical AI in all aspects.
Composable Data and Analysis: With data migration to cloud environments, the concept of composable data and analysis gains significance as a primary approach to creating analytical applications. This involves using multiple data, analysis, and AI solutions to enhance connectivity between data and business actions, creating more flexible and user-friendly experiences. According to Gartner, this strategy boosts productivity, speed, collaboration, and analytical capabilities.
The Rise of "Data Fabric": The concept of "data fabric" represents an architecture that encompasses various data services in environments ranging from local to cloud. This approach integrates and combines data management across different locations, significantly reducing integration, deployment, and maintenance times.
Focus on Small and Diverse Data: Gartner anticipates that the future of data will rely on the progressive use of smaller and more diverse data. Unlike "big data," these smaller and varied data sets enable complementary analysis and use of multiple data sources, whether structured or unstructured. Small data is valuable because it requires less information to yield useful results, allowing organizations to reduce their data assets.
The Era of XOps: More and more companies are adopting XOps, a set of business operations that includes data, machine learning, models, and platforms. This optimizes DevOps practices, scales prototypes, and provides more flexible designs. XOps technologies automate operations and enable the construction of data-driven decision-making systems to enhance business value.
Designed Decision Intelligence: Decision intelligence involves the use of technologies like data analysis, artificial intelligence, and APIs of complex adaptive systems for informed business decision-making. This architecture speeds up the acquisition of necessary information to drive business actions. Combined with composability and a common data fabric, designed decision intelligence opens new opportunities to optimize decisions and make them more precise, repeatable, and traceable.
Data-Centric Approach: Implementing a data-driven culture is essential for long-term business productivity. The trend is to place data at the center of business actions, strategies, and decisions, rather than being a secondary focus. The incorporation of a Chief Data Officer in defining strategies and decisions can significantly increase continuous production of business value.
Growing Importance of Data Visualization: Data visualization and graphs continue to be essential for discovering relationships between data assets, democratizing information, and improving decision-making. Gartner predicts that by 2025, 80% of innovations in data and analysis will use graph and visualization technologies.
From Passive Consumers to "Augmented Consumers": Predefined dashboards and manual data exploration will be replaced by automated, mobile, and dynamically generated information. Entrepreneurs will cease being passive consumers of data, thanks to new information formats tailored to their needs.
Rise of Edge Computing: Edge computing continues to expand, bringing data, analysis, and data technologies closer to the real world. Gartner forecasts that by 2023, over 50% of the data used by data analysts and scientists will be created, processed, and analyzed in edge computing environments.
In summary, business transformation is ongoing, and the Covid-19 pandemic has accelerated this evolution. The presented changes can be viewed as opportunities for digitalization and business innovation.
Data Maturity Model
In the digital era, managing data effectively is crucial for companies seeking to maintain their competitiveness. Data maturity reflects an organization's ability to handle and utilize its data effectively, directly impacting decision-making and operational efficiency.
What is the Data Maturity Model?
The data maturity model is a tool that allows organizations to assess and improve their data management capabilities. This model is based on the evaluation of different key areas, providing a roadmap to advance from basic data management levels to advanced integration and optimization.
Benefits of Evaluating Data Maturity
Evaluating a company's data maturity level helps identify areas for improvement and establish a concrete action plan to progress. This not only enhances the quality and accessibility of data but also facilitates the implementation of advanced technologies such as artificial intelligence and predictive analytics.
Practical Guide: Data Maturity Model
Bismart offers a downloadable guide that helps companies assess their data maturity level. This tool provides a detailed diagnosis and specific recommendations for each stage of the maturity model.
By understanding and improving data maturity, companies can optimize their operations, make more informed decisions, and remain competitive in a constantly evolving digital environment.
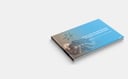
Guide: Data Maturity Model
Find out what level of data maturity your company is at with our guide for CDOs and data officers.