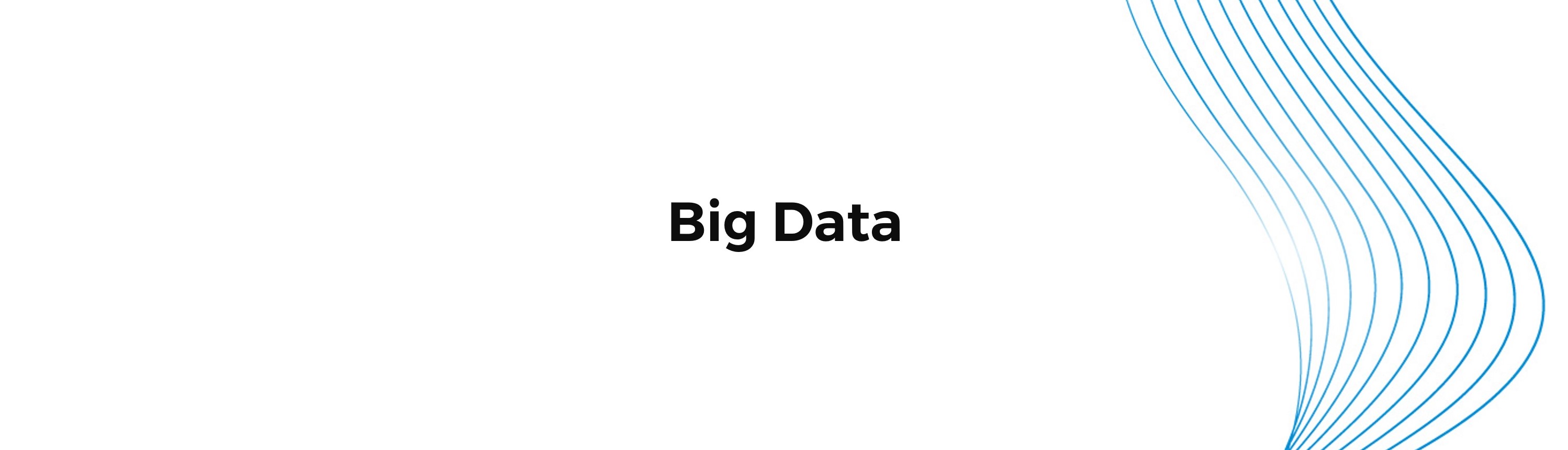
Big Data Analytics
Big data is the large volume of data, both structured and unstructured, that can help companies improve their operations and make smarter and faster business decisions. This is achieved by analyzing this data, which contains valuable information and patterns that cannot be found by analyzing it manually or even with traditional software and platforms. There are still many companies that have this data, but do not use it because they are unaware of the informational value hidden in it that can serve as the basis for informed and strategic business decisions, known in the business world as data-driven decisions.
When collected, this data is formatted, stored and analyzed, and its analysis will provide the company with very interesting information that will help it to increase revenue, explore and gain loyal customers.
What is Big Data Analytics?
Big data analytics is a business intelligence and data analysis service used in many industries that helps companies and organizations make better business decisions based on their big data.
Big data analytics offers, among other things, the ability to create a more effective marketing strategy, new revenue opportunities, better customer service, improved operational efficiency and competitive advantages over rival organizations from the information extracted from big data.
Many companies in different industries, such as insurance, banking, automotive, healthcare, etc., use big data analytics. These companies adapt the data for their businesses and departments (marketing, operations, finance, IT, etc.).
Thanks to the development of big data, companies and businesses have become more efficient and profitable because they can analyze in advance all the information and data they may need about their customers and potential customers, while optimizing their relationships with different sites and proposing alternatives to improve them.
Why is big data analytics useful?
Big Data analytics is useful because it allows companies to find answers to many questions: how to reduce costs and time, what new products to develop, smart decisions and process optimization. The combination of Big Data with powerful analytics is an opportunity and a possibility for companies to create value.
By using Big Data Analytics, you will have greater control of data resulting in more accurate analysis. Decision making can simplify operational performance, minimizing risk, and ensuring cost reduction.
We can define Big Data Analytics as a new competitive advantage. Its use is becoming essential for companies to perform to the best of their capabilities.
Companies also use Big Data Analytics to respond quickly to the needs of their customers, treating their potential customers individually, thus achieving happy customers, and long-term relationships. Thus, they become loyal customers to the company.
Companies also use Big Data Analytics to respond quickly to the needs of their customers, treating their potential customers individually, thus achieving happy customers, and long-term relationships. Thus, they become loyal customers to the company.
What are the advantages of big data analysis?
1. Better and faster decision making
Big Data Analytics has always involved trying to improve decision making. Large companies are looking to be able to make faster and better decisions with Big Data analytics, and they are succeeding.
2. Cost reduction
Based on the company's results, profits and return on investment. In short, the use of Big Data Analytics enables better performance and lower costs.
3. New products and services
The most interesting use of Big Data Analytics is undoubtedly the creation of new products and services for customers. Online businesses have been doing this for many years. Now with Big Data Analytics, even large traditional businesses are creating an increasingly complete offering for their customers' satisfaction.
To analyze Big Data, Microsoft recommends the use of Power BI for Office 365, a program that works as a set of business analysis tools, with the aim of analyzing data and sharing information. This tool can monitor the company's activity and respond quickly with comprehensive dashboards available on any device.
This is an analytical solution that helps any organization willing to control its activity through interactive reports. If you want to know more about Power BI, just talk to us!
The challenge of Big Data Analytics is to use this data to achieve new business objectives that were previously impossible to achieve. A few years later and thanks to technological advances, this quantitative change we have faced has also led to a qualitative change.
Data storage
A data warehouse is a data store. It can be a physical or logical warehouse and collects data from many sources to be analyzed and queried. Data warehouses are hosted on company servers or in the cloud.
It is a storage architecture that enables the organization, understanding and use of data for strategic decision making. It is much more than just a data warehouse. A data warehouse stores the data needed for reporting, analysis and other business analysis functions.
That is the very difference between a data warehouse and a database. Databases are limited to collecting information, while data warehouses have analysis capabilities on that information and are designed on the basis of OLAP systems to aggregate data for analysis.
The fact of being able to centralize information and combine historical records with current data makes it possible to enrich reporting, since reports are then drawn up on the basis of data from many different sources. This also makes it possible to discover patterns and trends and provides a rapid response capability. A data warehouse makes it possible to have all the information in one place, thus increasing efficiency.
The implementation of a data warehouse is necessary when the volume of data generated in the company is significant. It is important to have a good management plan and leave nothing to chance or improvisation. This can minimize risk, as traditional methods are designed to work with a fixed amount of data that can cause agility problems if exceeded.
In addition, having a centralized warehouse allows data quality control, since working with independent warehouses can generate duplication and negatively influence data quality issues.
What do we do at Bismart? - Data warehousing and business analytics
At Bismart, as a preferred Microsoft Big Data partner and Power BI partner, we work on the analysis and processing of Big Data to achieve a comprehensive and integrated view of our customers. In addition, we offer data warehousing and data processing services fully adapted to your specific needs. Our proposal differs from others by the following factors:
- Data quality: as we have already explained, data quality is essential in a data warehouse. This is why data quality is a priority in our projects.
- The data model: we strive to make the data models simple and to make the names of the entities and fields easily understandable for the users and to avoid any confusion about their meaning and purpose.
- The presentation of the reports: we take care of all the details of the presentation of the reports so that they are attractive, interactive, simple and usable. We always adapt them to the company's style and corporate image and advise them on the use of the most appropriate visual objects.
Data warehouse for strategic decision making
The data warehouse is linked to the concept of big data and business intelligence. Many companies use them to perform analysis and thus have a clear view of their business to make better decisions.
The data stored in a data warehouse consists of structured and unstructured data. It can come from many sources within the company or it can be external data that allows to generate a more complete view of the situation. For example, a transport company can add, in addition to its own data, traffic data in order to make decisions on the creation of new lines or to solve problems. These data must be processed, standardized and standardized so that they are accurate and of high quality and can be used to support decision-making.
For the sales manager, the data warehouse is an unseen foundation that must exist and that guarantees that the data obtained in the reports are correct. It is difficult to calculate the ROI of a data warehouse, but what we can foresee are the catastrophic effects that can be generated by having errors in the stored data. At Bismart we believe that strategic decisions should be based on data and not on assumptions. For this a great ally is a data warehouse.
How is Big Data used in real life?
Big Data is everywhere. More and more companies are finding new and interesting ways to leverage data and technology to offer fun or informative products and services. While Big Data is useful for solving many important problems (such as medical reports, artificial intelligence, communication services, etc.), companies are bringing out their creative side to use it for a wide variety of things. Today, algorithms can process Big Data to improve consumers' lives in very interesting and innovative ways. Here are some of them:
Spotify
Spotify is one of the companies that has really taken advantage of Big Data. They use many innovative data techniques to revolutionize the consumption and enjoyment of music. The company, with over 100 million users, runs entirely on data. They use intelligence on factors such as how long songs are played, where they are listened to, when, and on what type of device. All that information provides the music industry with fascinating insights that can make an impact on the listener experience.
Spotify recently released Spotify for Artists, which gives artists and their management access to data to improve their marketing and content. Spotify also uses Big Data for its "fans first" initiative. That allows them to offer their most dedicated fans access to special offers on concert tickets, merchandise, singles and more.
eHarmony
Dating companies like eHarmony use Big Data to increase their users' chances of finding love. Some of the statistics they use are surprising. In the past, dating sites used surveys or personality tests to determine each person's preferences. Then, they used algorithms to match people who had a high percentage of getting along.
Now, with the addition of Big Data those same companies can use more detailed factors to create matches with a future. Wearable devices can collect data such as sleep patterns, heart rate and physical activity levels. Those are all important factors when choosing the perfect match. Even if someone says they are "very active" or an "early riser," dating apps can check their actual data. That allows them to not only determine a match's suitability, but also to see if they're honest about their habits. Then, they use that data to generate better matches.
Snow Leopard Trust + Nature Conservation Foundation
Another very interesting way of using Big Data is to save rare animals by tracking and catching poachers. It is being used, more specifically, in India, to save tigers.
Poachers move in small nomadic groups and track their prey, making them difficult to catch in India. Tiger bones are very valuable for traditional Chinese medicine, so Indian tigers are worth a lot of money. The Snow Leopard Trust and the Nature Conservation Foundation worked together to combat the problem. They collected and analyzed data, from the most recent to data from 1972, from 605 districts in India. By analyzing Big Data they can predict where and when poachers are most likely to act.
Better yet, using handheld trackers, conservationists can collect more data. That allows them to target more specific tracking in the future. That's just one example of how Big Data can be used to fight crime and poachers.
Medical research
The current President of the United States, Joe Biden, announced in 2016 - at the time Vice President of Barack Obama's administration - that the Obama administration had created a multi-million dollar cancer research project using Big Data. The project, Cancer Moonshot Initiative, was born with the goal of leveraging massive data collection and processing to find a cure for cancer in the next decade.
The combination of Big Data and artificial intelligence has given rise to such interesting technologies as folksonomy. Folksonomy, unlike taxonomy, is a system that takes advantage of artificial intelligence and machine learning capabilities to recognize patterns in large amounts of textual data.
Bismart has its own folksonomy solution: Folksonomy Text Analytics, presented at the Big Data Congress 2017. We have collaborated with a number of well-known healthcare companies, which have applied folksonomy to analyze large amounts of data on the evolution of patients related to specific diseases.
This 2020 and 2021 Bismart has initiated a collaborative project the Hospital del Mar and Laboratorios Ferrer to analyze the clinical situations of COVID-19 patients, to be able to make predictions and study the evolution of the virus and its effects on the human body. The basis of the project was presented at the symposium "Covid-19 clinical research: challenges and innovation."
Bismart cuenta con su propia solución de folksonomía: Folksonomy Text Analytics, presentada en el Big Data Congress de 2017. Hemos colaborado con númerosas y reconocidas empresas sanitarias, que han aplicado la folksonomía para analizar grandes cantidades de datos sobre la evolución de los pacientes relativa a enfermedades concretas.
Bismart has also applied Folksonomy Text Analytics to the nephrology team at Hopspital del Mar, led by Dr. Sans.
Society
Big Data offers the possibility of improving the living conditions of people at risk of social exclusion and energy poverty. This requires the standardization of databases and tools to process the data obtained. This will help those families who do not actively seek help, as well as empowering them to live off their own resources. Technology provides the ability to take immediate action, ongoing care and improved resource management. This would help improve a person's quality of life and fight poverty, so it is important to invest in these resources to help families.
On the other hand, Big Data solutions are also being applied to improve the lives of the elderly. The elderly population is increasing and countries around the world are facing this reality. The increase in the age of the population is already causing changes in society. For this reason, Big Data solutions are needed to address this change. Big Data solutions can help predict which seniors will need more care, protect them from financial fraud, and monitor their health. These solutions must be tailored to the specific problems of seniors. Together, Big Data can help seniors around the world.
Magic Mirror
Big Data can also be used to create a whole new experience for shoppers. Our Magic Mirror not only works as a regular mirror, but it also gives shoppers personalized recommendations for pieces they may be interested in. It can analyze their expression, mood and personal style to give them that information.
On top of that, Big Data correlates their personal shopping history with their preferences, socio-demographic information, etc. The mirror offers them the perfect product that they won't be able to resist buying. You can read more about Magic Mirror in our previous post.
Gaming
Big Data is great for games. Are you familiar with the "Choose Your Own Adventure" books? Well, now, with Big Data and artificial intelligence we can interact directly with plots that will anticipate how we will make decisions. For example, we will be able to play chess with a computer that constantly learns from our moves. The computer gets smarter with each move and remembers previous games.
That's great for training in various areas, but from a more fun perspective, Big Data is used to make video games more and more difficult. You can practice playing your favorite board or puzzle game with your computer and imagine how good you will be the next time you play with your friends.
Public Administration
Big Data has become a fundamental tool for public administration, as it manages to convert large amounts of data (Big Data) into valuable public information.
Public administration agencies work with a large amount of information, stored in any agency and in multiple data sources. And of course, in unstructured forms. Big Data is no longer a common phenomenon in public administration.
The tools and treatment of Big Data greatly facilitates the work of public administrators and manages to optimize the management of public resources and lighten processes that are usually extremely slow.
Maps on mobile and GPS
Remember when we had to look at maps before going on a trip? Many of us had them folded up in the glove compartment of the car for when we inevitably got lost on a trip to the mountains or the beach. Then we had MapQuest: before embarking on a trip with more than one stop, we would meticulously plan each stop in advance, making sure to print out the appropriate directions. Finally, thanks to satellite GPS technology, we were able to enter the departure point and destination from anywhere and receive real-time instructions.
Today, Big Data enables the continuous improvement of mobile maps. As the Internet of Things is something that gets better the more it is used, with the introduction of more mobile apps and more smartphones and the liberalization of telecommunication networks, the amount of Big Data being used to provide concrete directions in real time is staggering.
If you go into Google Maps on your smart device you will see that the directions are getting better and better and more detailed with options for cyclists and, in some cases, public transport. The most developed Smart cities even provide intermodal traffic routes that are faster for getting between two specific points.
Online shopping
Since the growth of Big Data in the retail industry, the industry is changing completely. Retailers are using Big Data from the moment you start searching from targeted ads to the delivery of your order. In Amazon's case, even leaving the package inside your home with the new Amazon Key service. Of course, Big Data is present in online shopping: search engines take note of your trends, maybe even with GPS, to bring you the ads that might interest you the most.
The online profile of a buyer only gives the seller a fraction of the information that will allow him to optimize his offers. By tracking click-throughs, the seller can begin to form a substantially more useful buying profile. That, along with demographic and location information allows the seller to consolidate massive amounts of data from other buyers through complex event processing, enabling dynamic segmentation and online sales success.
Big Data also affects real-space commerce. A great example is Nordstrom's new concept. Nordstrom Local is a store that uses Big Data technology to minimize retail space: a store that sells nothing. Business Insider explains that the store will only be about 280 square feet and will consist of fitting rooms. Personal stylists will pick up products from other Nordstrom spaces or through Nordstrom's website.
That's possible because thanks to Big Data we can get fast and concrete transportation of goods in real time. Customers can buy online, go to the store, try it on, and finally finish the purchase. They can choose to take it by hand or receive it at home.
As space is becoming more and more expensive, Big Data can be used to come up with new and creative sales models in the near future.
Urbanism
Big Data is constantly being used in the context of smart cities to plan urban centers. Big Data enables urban planners to develop new insights into the functioning of cities much more quickly than before. Urban planners design cities in minutes, hours or days, rather than over the course of years or decades. A good example of Big Data in urban planning is the use of data to improve public transportation performance.
Subway transportation systems can now track passenger flow in real time. They can do this with ticket sales and validation systems when users enter or exit the train. Transit operators can use that information to determine peak hours. They can then adjust the number of cars available on the tracks in certain areas at specific times. Delays or accidents can also be reported and communicated to passengers in real time, so they can plan their travel plans. This is especially useful in cities where large events are held regularly.
On the other hand, to guarantee citizens' privacy, cities and local administrations need to ensure that their information systems comply with data anonymization standards. This means that personal data collected cannot be used to identify a specific person. It is also important to ensure the quality of the data collected, ensure data homogenization and geo-referencing. This will allow cities to collect accurate and relevant data for decision making. Finally, to ensure interoperability between proprietary and third-party information systems, it is important to create a database of high quality data that can be automatically populated. This will enable cities to improve the management of citizen data and ensure that public services are more effective.
Tourism
Tourism is an important global economic sector that generates around 10% of the world's GDP and offers great potential for wealth and employment. Advances in Big Data technologies applied to tourism allow cities and tourism boards to evaluate traditional and new indicators to improve tourism management. This translates into personalized offers for tourists, as well as improvements in the quality of tourism services. The UN has focused on how to measure and create more sustainable tourism for both suppliers and customers.
Power consumption
Big Data enables smart meters to autonomously regulate energy consumption for more efficient use. Smart meters collect data from sensors scattered throughout the urban space. They determine when there is a higher or lower flow of energy, just as transportation planners do with people. The energy is then redistributed throughout the grid to go to the places with the highest demand. Smart meters may be expensive in the short term, but they will be the new norm. They will automatically adjust to ensure the smart distribution of energy across the respective grid.
Portable devices
Finally, wearable devices are everywhere. Wearing a device that monitors our physical activity and sleep is great for keeping track of our health and fitness. We used to have to write everything down to remember what we ate or how far we walked. Now with technology changing our lifestyle habits, we can track ourselves to make sure we maintain healthy habits.
In the 1980s and 1990s we witnessed a big shift from people working in factories to people working in offices. That also meant an alteration in our movements and diet. Most Westerners are more sedentary than ever in comparison. Wearable devices provide technology to monitor ourselves and use that data to provide valuable feedback for continuous improvement.
Other ways in which Big Data is changing the world
At Bismart we are experts in using Big Data solutions to solve real-life problems. Right now, countries around the world are facing a real problem: traffic accidents. European countries are showing particular interest in this issue because of the European Union's Road Safety Program, which sets ambitious targets to reduce road accidents.
Current situation
Road accidents are serious business. In 2011 alone, more than 30,000 people died on the roads in the European Union, the same number as live in a medium-sized municipality. And for every one of those 30,000 deaths:
- 4 people were permanently disabled
- 8 were severely damaged
- and 50 suffered minor injuries.
Until 2015, the trend in the number of traffic fatalities was a constant downward trend. However, the trend has since reversed.
The problem is that the organizations trying to reduce traffic accidents do not have enough resources to lower the accident rate, whether it is adding more controls or more personnel. Local departments are overwhelmed; they can't use more resources.
Conventional solutions have done what they can. If we really want to reduce traffic accidents, it is imperative that we turn to technology.
While these organizations can no longer implement their techniques, there is a powerful tool they can use: Big Data solutions.
Not only are these solutions better than current ones, but they can significantly reduce traffic fatalities for a much lower price, according to Smart Cities World.
How can Big Data solutions increase road safety?
Many cities are implementing innovative Big Data projects to increase road safety. In Europe, there are programs led by the European Union and companies working on ways to use technology to reduce traffic accidents.
The EU has designed a strategy to create cooperative intelligent transport systems, which focus on reducing human error, optimize transport systems and create environmentally sustainable solutions. They also stress the need to exchange data between different stakeholders in the transport system, a frequent problem that organizations must deal with when implementing Big Data solutions.
Another EU-led project is the Commission's GEAR 2030 initiative, focused on boosting automated driving and connected vehicles.
In addition to EU initiatives, many companies are developing creative ways to increase road safety.
One of them is the British company INRIX, which uses Big Data to provide safety warnings, which inform the driver when they should slow down due to dangerous situations on the road. It can also give speed estimates.
Bismart's Traffic Fatalities Prevention solution goes one step further. It not only analyzes how we can improve safety, but also provides prescriptive recommendations with concrete steps organizations can take to reduce accidents.
Other uses of Big Data
Big Data has become an increasingly popular tool for improving business decision making. This is because Big Data helps companies store and process large amounts of data so they can gain insights from the data and make more informed decisions. Big Data can also help companies improve operational efficiency, reduce costs, improve innovation and productivity, and improve customer satisfaction. In addition, Big Data can be used to improve information security, market analysis, project management, and fraud detection. In short, Big Data is a useful tool for companies to help them improve their operations and make better-informed decisions.
Big Data in companies
Data is already one of the most important assets for companies. However, the real use of data and obtaining value from Big Data requires the implementation of a data-driven culture in the organization.
In other words, the Big Data culture must be part of a company's DNA. Without a prior change in the cultural model of companies, companies will remain data-driven and unable to get the most out of their data assets.
According to Philip Carnell, Research Director at IDC European Software Group, 94% of companies agree that Big Data has great value in supporting their operations and business objectives. However, only 35% of companies surveyed by IDC invest in data analytics tools.
But what do companies need to do to be data-driven?
First of all, it is essential to consider the wide range of possibilities of cloud infrastructure. Cloud servers have many advantages over on-premise, not the least of which are greater speed, scalability, capital savings and lower maintenance cost investment. While in 2017, 73% of companies were already using cloud services or were considering doing so, by 2021, the figure rises to 89%.
On the other hand, it is necessary to adapt to the new security and data protection laws. The new European legislative model for data protection, the GDPR (General Data Protection Regulation), has revealed that companies are still not doing their homework on data governance and data quality.
Beyond what we are still doing wrong or, rather, failing to do, Big Data companies are a great job opportunity for many reasons. For starters, right now data analytics plays a critical role in any enterprise. Moreover, data, data science, data analytics and statistics are the key to making informed business decisions. For another, data analysts and data scientists have the great advantage that they are needed in all industries and types of business and therefore can work in any business sector.
In short, working in Big Data is working in the future.
The best Big Data tools for 2021
Beyond the implementation of a data-driven culture and qualified personnel, organizations require specialized tools that allow them to process massive amounts of data in order to transform their data assets into value and leverage them to optimize their business processes.
Today the market is full of tools and technologies specialized in data processing and analysis. However, not all of them have the capacity to process large amounts of data. Also, as the offer of Big Data tools expands, choosing the right tool according to our business needs becomes more complex.
In this sense, when acquiring one tool or another, organizations must take into account aspects such as technical capabilities, maintenance, usability of the application, etc.
Below we list what, according to our team of experts, are the 8 best Big Data tools of 2021 and specify what each one is for.
Data Lake Tools
Data lakes are a type of data storage repository that stores data in its original format; intact, until those in charge of handling the data decide on its use within the organization. The differentiating aspect of a data lake is that, unlike other types of data repositories such as a data warehouse, it has a flat architecture and does not organize data assets into files or folders.
1. Azure Synapse
Azure Synapse is a complete cloud data processing, transformation and treatment service. If you have been working in the data field for some time, you probably know that Azure Synapse used to be called Azure SQL Data Warehouse. After an update and name change, the new platform includes new capabilities such as integration with Apache Spark or the ability to store and analyze large amounts of data with a single platform.
2. Azure Databricks
Azure Databricks is another Microsoft tool that stands out for enabling version automation, real-time co-authoring and machine learning capabilities.
3. Snowflake
Snowflake is a multipurpose cloud tool that can be used both to store data and to carry out data science or data engineering processes. You can also use this tool to develop APIs.
4. AWS
Amazon Web Services (AWS) are another essential Big Data tool. Its service for storing large amounts of data, Amazon Redshift, incorporates great scalability possibilities and decreases data loading time thanks to its massively parallel processing.
Data integration tools
Data integration is another basic and essential process for any company. Thus, data integration tools are very useful for businesses.
5. Azure Data Factory
Azure Data Factory is a data integration service in the cloud from Microsoft. This platform enables the integration of multiple data sources and the possibility of transforming them to adapt them to the business objectives of each company.
Its most outstanding capabilities are the possibility of creating workflows and that the tool incorporates more than 90 connectors that allow companies to integrate cloud platforms with on-premise platforms.
6. Informatica
Informatica is another of the most popular data integration tools. Its specialty is Big Data collection and processing, as it manages large amounts of data from anywhere. It also includes data analysis capabilities.
Data visualization tools
Data visualization is another essential factor in transforming large amounts of data into digestible and useful information.
7. Power BI
Power BI is a set of BI platforms specially designed to serve the business objectives of organizations.
Among the most prized capabilities of this tool are its great data visualization capabilities. Power BI visuals are among the best on the market and, in addition, it allows non-expert users to create effective visualizations thanks to its great usability. If we compare Excel with Power BI, for example, Power BI is definitely easier to use than Excel.
Power BI is one of the best data visualization tools on the market and allows companies to easily create both reports and dashboards.
Interested in learning how to develop better reports and dashboards in Power BI? Download our e-book with the 21 best practices for creating reports in Power BI!
8. Looker
Looker is another valid option for designing data visualizations and analyzing data sets. This Google tool has its own coding language: LookML; thing that makes it less accessible and less integrable with other tools.
Big Data and ETL
The transformation of ETL (Extract, Transform and Load) processes by Big Data is a phenomenon that has completely changed the way these tasks are performed.
Big Data provides a powerful platform for ETL that offers many advantages over traditional approaches. For example, Big Data enables much more agile processing, offering greater scalability and improving efficiency. This is because Big Data enables distributed processing across a variety of compute nodes, meaning that data can be processed simultaneously in parallel. This also enables the processing of larger and more voluminous data. In addition, Big Data enables in-memory processing, which means that data can be processed much faster than with traditional approaches.
Big Data also offers greater flexibility for ETL processes. Big Data tools offer a wide variety of options for extracting, transforming and loading data. This means that users can leverage Big Data tools to create a customized workflow to meet their specific data needs. This means that users can use Big Data tools to perform tasks such as data extraction and cleansing, data transformation, data integration and analysis, as well as reporting. This means that ETL processes can be automated much more effectively and efficiently.
In short, Big Data has transformed ETL processes from a traditional server-based approach to a much more agile, flexible and scalable cloud-based approach. It offers a variety of tools that allow users to control their data and perform ETL tasks more efficiently and quickly. This has enabled organizations to better leverage the information available.
Big Data, data compliance and GDPR
The General Data Protection Regulation (GDPR) is a new European legislation that applies to all companies that collect, store, process and analyze personal data of European Union citizens. The Organic Law on Data Protection (LOPD) is a Spanish law that regulates the collection, use, storage and distribution of personal data of Spanish citizens.
These laws exist to protect the privacy of individuals and to ensure compliance with international data protection standards. In today's world, the collection, use and storage of personal data has become increasingly common. This is due in part to the rise of Big Data technology.
Big Data technology is used to collect, store and analyze large amounts of personal data. This enables companies to make better business decisions, improve products and services, and learn more about their customers. This also raises important concerns about data privacy and security.
The collection, use and storage of personal data have become increasingly common due to the rise of Big Data technology. This technology is used to collect, store and analyze large amounts of personal data, enabling companies to make better business decisions, improve products and services, and learn more about their customers. However, this also raises concerns about data privacy and security. Therefore, the GDPR and LOPD laws establish the rights of citizens in relation to their personal data and set out requirements for the protection of their personal data.
How can you introduce Big Data analytics in your company?
It's all the fault of Big Data. We are capable of creating, storing and accessing a huge amount of data, but we don't always know how to read it, relate it and use it to our advantage. The solution is not only to choose the right Business Intelligence tools, but also to build a strategy for our organization to adopt the data culture.
Download our Whitepaper on Big Data and discover what your data can do for you.
Evolution of data integration processes: From ETL to ELT driven by Big Data
The advent of Big Data has revolutionized data management and storage, generating new demands on traditional storage processes. Over time, the increasing requirements in terms of volume and velocity have led to an evolution in ETL processes, adopting a different perspective known as ELT.
For more than four decades, the ETL (Extract, Transform and Load) process has been the conventional method for integrating data from various sources into a single data warehouse. This approach involved extracting data from the source sources, transforming it into a temporary database and finally loading it into the enterprise data warehouse. ETL worked well when the data warehouse was a relational database with predefined schemas, and has been widely used with a variety of ETL tools in a multi-billion dollar market.
However, new demands in terms of volume, speed and veracity in data integration and storage have led to a different approach. ELT (Extract, Load and Transform) has emerged, which alters the traditional order of the ETL process. In this new approach, data is extracted from source sources and loaded directly into the target data warehouse. Unlike ETL, data transformations are performed in the target data warehouse itself, as needed.
So what has happened to ETL, why has ELT emerged, and is the ETL process obsolete?
In the following, we will try to answer these questions.
The term "Big Data" emerged in the late 1990s to describe the issues facing organizations. In 1997, a group of NASA researchers published a paper noting that "the growth of data was becoming a problem for today's computer systems." The exponential growth of data spurred technological advancement toward platforms capable of handling massive data sets. In 2001, the US firm Gartner published research called "3D Data Management: Controlling Data Volume, Velocity, and Variety", which mentioned for the first time the "3Vs" that define Big Data technologies: volume, velocity, and variety.
Big Data posed challenges for the ETL process. The volume, velocity and variety demanded by Big Data challenged the performance of ETL tools, which were often unable to handle the pace required to process large volumes of data due to capacity and velocity constraints, as well as additional costs.
The emergence of new data formats and sources, as well as data consolidation requirements, revealed the rigidity of the ETL process and changed the usual way of consuming data. The demand for greater speed and variety led data consumers to need immediate access to raw data, rather than waiting for IT to transform it and make it accessible.
On the other hand, Big Data also drove the emergence of the data lake, a data warehouse that does not require a predefined schema, unlike the traditional data warehouse, which introduced more flexible storage schemes.
ETL tools, which were traditionally designed to be managed by the IT department, are complicated to install, configure and administer. Moreover, these technologies conceive data transformation as a task exclusively for IT experts, making it difficult to access for data consumers, who, according to ETL logic, should only be able to access the final product stored in a standardized data warehouse.
As is often the case, context drove innovation. ELT presents itself as the natural evolution of ETL, reshaping the process and making it more suitable for working with Big Data and cloud services by providing greater flexibility. It also facilitates scalability, improves performance and speed, and reduces costs.
However, ELT also has its own challenges. Unlike ETL, ELT tools are designed to facilitate data access for end consumers, which democratizes data access and allows users to access data from any data source via a URL. However, this can pose risks to data governance.
From a business perspective, getting rid of all ETL investments and technologies to completely migrate to ELT would not be cost-effective. On the other hand, companies that have not yet invested in either process should evaluate their data integration needs and decide which approach best suits them.
In addition to data governance considerations, ELT also presents other contradictions. Although it optimizes both extraction (E) and loading (L), it still faces challenges in terms of transformation (T). Data analytics plays a key role in businesses today, and despite the efforts of this new approach, data transformation, which is necessary for analytics, has not been simplified and remains the domain of the IT department, particularly data engineers and data scientists. In addition, transforming raw data into consumer-ready assets still requires multiple tools and complex processes, which data consumers do not have the capacity to address. In addition, data transformation still faces challenges in terms of process speed, allocation of required resources, cost and scalability, similar to those faced in the ETL approach.
Is the problem solved? For ELT to definitively replace ETL, ELT tools must evolve. It is expected that in the near future, these tools will include data governance capabilities and will gradually solve the remaining drawbacks. Some experts propose a solution that introduces a new twist in the history of data extraction, transformation and storage: EL+T, combining elements of both approaches.
In conclusion, the future of data extraction, loading and transformation is still evolving, and companies should carefully evaluate their needs and consider both ETL and ELT, as well as possible hybrid solutions, in their quest for efficient data management.
How can we help you?
Bismart's solutions, based on Microsoft Power BI business analytics applications, will help you transform your data into knowledge through Big Data management, Dashboards, Key Performance Indicators, Data Mining, Reporting, Data Warehousing and Data Integrations.
Through intelligent algorithms created by our experts, we customize your case and help you build a data-driven strategy to increase the knowledge of your customers, the efficiency of your marketing campaigns, optimize your processes, train professionals or discover new business opportunities.