Case Study: Development of a RAG Intelligent Search Engine
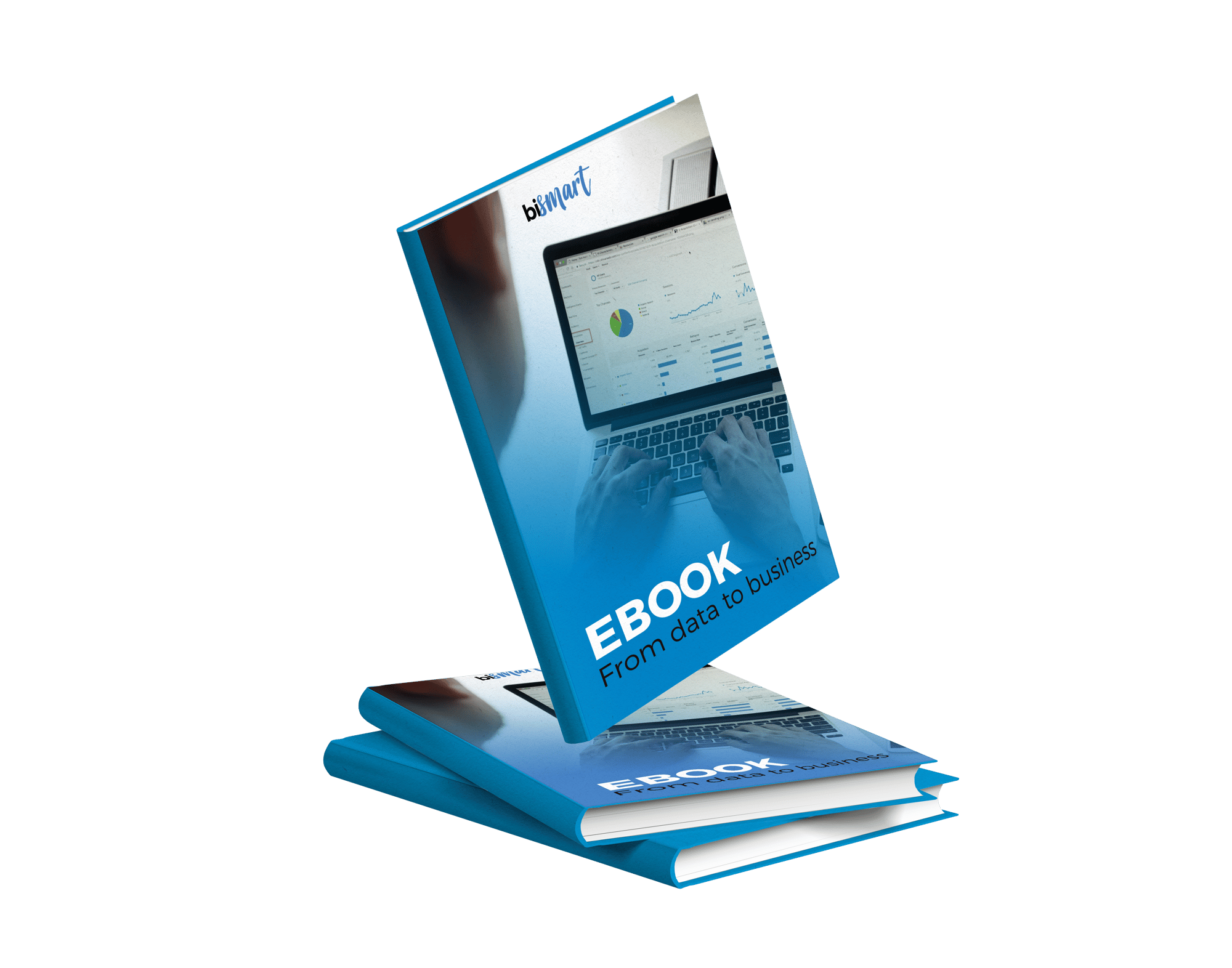
▶️ Discover how intelligent automation revolutionized the management of court notifications in our case study.
▶️ Learn how we implemented a RAG search engine to optimize complex processes, explained with concrete results.
▶️ Explore a practical example of how AI transforms the automation of legal tasks and information search.
What is a RAG Search Engine?
A RAG (Retrieval Augmented Generation) search engine is an advanced type of search engine that combines two key technologies:
-
Information Retrieval: The system searches and extracts relevant data from large knowledge bases or documents, as a traditional search engine would do.
-
Natural language generation: A generative AI, such as advanced language models, takes the retrieved information and presents it in a well-structured, coherent and personalized response, rather than simply listing results.
This type of search engine excels at processing and understanding both structured and unstructured data (such as documents, records, etc.), using semantic capabilities to perform more precise contextual searches. Rather than simply displaying textual results like traditional search engines, RAG search engines generate answers based on the exact context of the query, combining the best of search and content generation.
These systems are revolutionizing the way information is searched, especially in complex areas such as the processing of large volumes of documents, for example, in hospitals, law firms or corporations that need access to detailed information in an understandable format adapted to each query.
What Is a RAG Search Engine and Why Is It Disrupting Enterprise Search?
A RAG (Retrieval-Augmented Generation) search engine combines information retrieval with generative AI to answer natural language queries using internal documentation or specialized data sources. Unlike traditional keyword-based search, RAG systems understand context and generate precise, conversational answers.
Key Advantages of RAG Search Engines
- Natural language queries with semantic understanding
- Search by meaning, not just keywords
- Extract insights from unstructured internal data
- Ability to process large volumes of legal and technical documents
Real-Life RAG Use Case in Legal Document Management
A company managing thousands of legal notifications daily needed to automate how these were read, classified, and processed. Legal teams faced high volumes of variable-format notifications from multiple judicial bodies that required rapid and accurate information extraction.
Key Challenges Before Implementation
- Massive volume of daily legal notifications
- Variability in document formats
- Urgent need to identify court, parties, case type, and dates instantly
How the Intelligent RAG Search Engine Was Designed and Deployed
The solution was built using a Retrieval-Augmented Generation architecture based on semantic search and generative AI models via Azure OpenAI, fully integrated with internal platforms such as Kamaleon and the client’s backend.
Key Components of the System
- Vector-based semantic search engine
- Document ingestion and processing pipeline
- Secure integration with Azure and internal back-end systems
- Generative language model fine-tuned on legal content
- Natural language interface for querying internal documents
Business Outcomes: Speed, Precision, and Scalable Legal Automation
The implementation allows legal teams to instantly extract relevant information from documents, drastically reduce manual reading time, eliminate errors, and respond more efficiently to legal demands.
Main Benefits Achieved
- Full automation of repetitive legal document tasks
- Increased efficiency for legal departments
- Human error reduction in critical operations
- Real-time access to key legal data
- Faster response to judicial requests
Why This Case Matters for Enterprises in Other Sectors
This success story shows how generative AI and RAG search can be applied in any organization that deals with complex document management and requires fast, accurate information retrieval.
Industries That Can Benefit from RAG Search Engines
- Legal: Case law analysis, contracts, court notifications
- Healthcare: Patient records, clinical protocols
- Corporate: Compliance documentation, policies, internal reports
- Banking & Insurance: Claims processing, policy interpretation, regulatory texts
Download the Full Success Story and Explore the Potential of RAG + AI
This downloadable success story includes technical details, architecture, implementation steps, and outcomes. A practical use case that shows how to put generative AI to work in document-heavy environments.
🧩 RAG FAQ
❓ What is a RAG search engine?
A RAG (Retrieval-Augmented Generation) search engine combines semantic search and generative AI to provide precise, conversational answers from internal unstructured data.
❓ How is RAG used in legal processes?
It automates reading, classifying, and retrieving legal data from documents, such as court notifications, using natural language queries.
❓ What are the benefits of implementing RAG AI in a company?
It increases operational efficiency, eliminates manual tasks, improves accuracy, and speeds up decision-making in document-heavy workflows.